The overarching research focus of our group centers on leveraging materials informatics and data-driven methodologies to advance high-impact research in the realms of materials design and discovery. Our endeavors encompass not only the pursuit of fundamental scientific insights but also the provision of innovative solutions to address significant challenges spanning various fields.
Our current interests are directed towards several domains, including achieving carbon neutrality, enhancing fuel production, harnessing solar energy, advancing water treatment techniques, and developing cutting-edge energy storage solutions.
Our strategic approach involves the establishment of a robust infrastructure, which encompasses the development of automated virtual high-throughput screening tools, the construction of comprehensive databases, and the implementation of AI-driven workflows tailored to address specific research inquiries.
Recent work
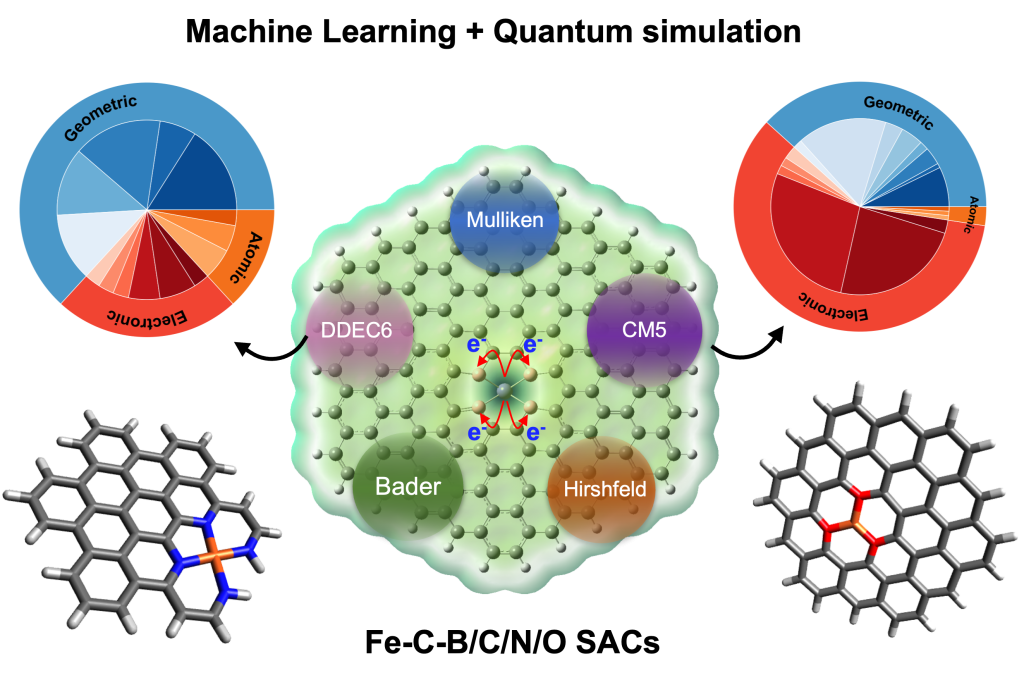
Can diverse charge schemes yield unified insights? Our study on Fe-centered SACs dives into Bader, Mulliken, Hirshfeld, CM5, and DDEC6 charges. Excitingly, DDEC6, Mulliken, and Hirshfeld show stronger correlations, impacting Fe’s atomic charge. Metal-substrate interactions sway these charges uniquely. Leveraging Quantum Simulations and Machine Learning, we predict charge schemes, revealing geometric and electronic influences. Discovering SACs’ design secrets, one charge at a time.